Revolutionizing Breast Imaging: Artificial Intelligence’s Role in Precisely Differentiating Benign from Malignant Lesions
Abstract
Recent advancements in artificial intelligence (AI) have leveraged computer science with large datasets to improve predictive and classification capabilities, which are crucial for problem‑solving in radiology. Machine Learning (ML), the driving force behind AI’s effectiveness, harnesses computational models and algorithms to analyze raw data for classification and prediction tasks. AI utilizes a multi-layered network of interconnected nodes emulating the intricate neuronal structure of the human brain. These include an input layer that initially receives data, a hidden layer that discerns data patterns, and an output layer that presents the results of the processed data.
References
Hu Q, Giger ML. Clinical artificial intelligence applications: breast imaging. Radiol Clin North Am. 2021;59(6):1027-l043. doi: 10.3322/caac.21552.
Pesapane F, Codari M and Sardanelli F. Artificial intelligence in medical imaging: threat or opportunity? Radiologists again at the forefront of innovation in medicine. Eur Radiol Exp. 2018;2:35. doi: 10.1186/s41747-018-0061-6.
LeCun Y, Bengio Y, Hinton G. Deep learning. Nature. 2015;521(7553):436-444. doi: 10.1038/nature14539
Sahiner B, Pezeshk A, Hadjiiski LM, Wang X, Drukker K, Cha KH, et al. Deep learning in medical imaging and radiation therapy. Med Phys. 2019;46(1):el-e36. doi: 10.1002/mp.
Mazurowski MA, Buda M, Saha A, Bashir MR. Deep learning in radiology: an overview of the concepts and a survey of the state of the art with focus on MRJ. J Magn Reson Imaging. 2019;49(4):939-954. doi: 10.1002/jmri.26534.
Yala A, Lehman C, Schuster T, Portnoi T, Barzilay R. A deep learning mammography-based model for improved breast cancer risk prediction. Radiology. 2019;292(1):60-66. doi: 10.1148/radiol.2019182716.
Dembrower K, Liu Y, Azizpour H, Eklund M, Smith K, Lindholm P, et al. Comparison of a deep learning risk score and standard mammographic density score for breast cancer risk prediction. Radiology. 2020;294(2):265-272. doi:10.1148/radiol.2019190872.
Yala A, Mikhael PG, Strand F, Lin G, Smith K, Wan YL, et al. Toward robust mammography-based models for breast cancer risk. Sci Transl Med. 2021;13(578):eaba4373. doi: 10.1126/scitranslmed.aba4373
Brunetti N, Calabrese M, Martinoli C, Tagliafico AS. Artificial intelligence in breast ultrasound: from diagnosis to prognosis-a rapid review. Diagnostics (Basel). 2022;13(1):58. doi: 10.3390/diagnostics13010058.
Liang X, Yu X, Gao T. Machine learning with magnetic resonance imaging for prediction of response to neoadjuvant chemotherapy in breast cancer: a systematic review and meta-analysis. Eur J Radiol. 2022;150:110247. doi: 10.1016/j.ejrad.2022.110247.
Davey MG, Davey MS, Ryan ÉJ, Boland MR, McAnena PF, Lowery AJ, et al. Is radiomic MRI a feasible alternative to OncotypeDX® recurrence score testing? A systematic review and meta-analysis. BJS Open. 2021;5(5):zrab081. doi: 10.1093/bjsopen/zrab081.
Oh KE, Vasandani N, Anwar A. Radiomics to differentiate malignant and benign breast lesions: a systematic review and diagnostic test accuracy meta-analysis Cureus. 2023;15(11):e49015. doi: 10.7759/cureus.49015
Lee JH, Kim KH, Lee EH, An JS, Ryu JK, Park YM, et al. lmproving the performance of radiologists using artificial intelligence-based detection support software for mammography: a multi-reader study. Korean J Radiol. 2022;23(5):505-516. doi: 10.3348/kjr.2021.0476.
Schaffter T, Buist DSM, Lee CI, Nikulin Y, Ribli D, Guan Y, et al. Evaluation of combined artificial intelligence and radiologist assessment to interpret screening mammograms. JAMA Netw Open. 2020;3(3):e200265. doi: 10.1001/jamanetworkopen.2020.0265.
Yoon JH, Kim EK. Deep learning-based artificial intelligence for mammography. Korean J Radiol. 2021;22(8):1225-1239. doi: 10.3348/kjr.2020.1210
Lee HJ, Nguyen AT, Ki SY, Lee JE, Do LN, Park MH et al. Classification of MR-detected additional lesions in patients with breast cancer using a combination of radiomics analysis and machine learning. Front Oncol. 2021;11:744460. doi: 10.3389/fonc.2021.744460.
Le EPV, Wang Y, Huang Y, Hickman S, Gilbert FJ. Artificial intelligence in breast imaging. Clin Radiol. 2019;74(5):357-366. doi: 10.1016/j.crad.2019.02.006.
Bahl M. Artificial intelligence: a primer for breast imaging radiologists. J Breast Imaging. 2020;2(4):304-314. doi: 10.1093/jbi/wbaa033.
Dabbous FM, Dolecek TA, Berbaum ML, Friedewald SM, Summerfelt WT, Hoskins K, et al. Impact of a false-positive screening mammogram on subsequent screening behavior and stage at breast cancer diagnosis. Cancer Epidemiol Biomarkers Prev. 2017;26(3):397-403. doi: 10.1158/1055-9965.EPI-16-0524
Nelson HD, Pappas M, Cantor A, Griffin J, Daeges M, Humphrey L. Harms of breast cancer screening: a systematic review to update the 2009 U.S. Preventive Services Task Force recommendation. Ann Intern Med. 2016;164(4):256-267. doi: 10.1158/1055-9965.EPI-16-0524.
U.S. Food & Drug Administration. Machine Learning (AI/ML)-Based Software as a Medical Device (SaMD) Action Plan. [Internet] US Food and Drug Administration [Published September 22, 2021, Accessed January 09, 2024] Available from: https://www.fda.gov/medical-devices/software-medical-device-samd/artificial-intelligence-and-machine-learning-software-medical-device.
Chan HP, Samala RK, Hadjiiski LM, Zhou C. Deep learning in medical imaging analysis. Adv Exp Med Biol. 2020;1213:3-21. doi: 10.1007/978-3-030-33128-3_1.
Haibe-Kains B, Adam GA, Hosny A, Khodakarami F, Massive Analysis Quality Control (MAQC) Society Board of Directors, Waldran L, et al. Transparency and reproducibility in artificial intelligence. Nature. 2020;586(7829):E14-E16. doi: 10.1038/s41586-020-2766-y.
Facciorusso A, Ferrusquía J, Muscatiello N. Lead time bias in estimating survival outcomes. Gut. 2016;65(3):538-539. doi:10.1136/gutjnl-2015-310199
Health Canada. Medical Devices Active License Listing (MDALL) - Your reference tool for licensed medical devices in Lead time bias in estimating survival outcomes Canada. [Internet]. Health Canada. [Accessed 2023 September 24, cited 2021 January 06]. Available from: https://health-products.canada.ca/mdall-limh/
U.S. Food & Drug Administration. Artificial Intelligence and Machine Learning (AI/ML)-Enabled Medical Devices. [Internet]. [Accessed 2022 September 24, cited 2021 September 22]. Available from: Artificial Intelligence (AI) and Machine Learning (ML) in Medical Devices (fda.gov)
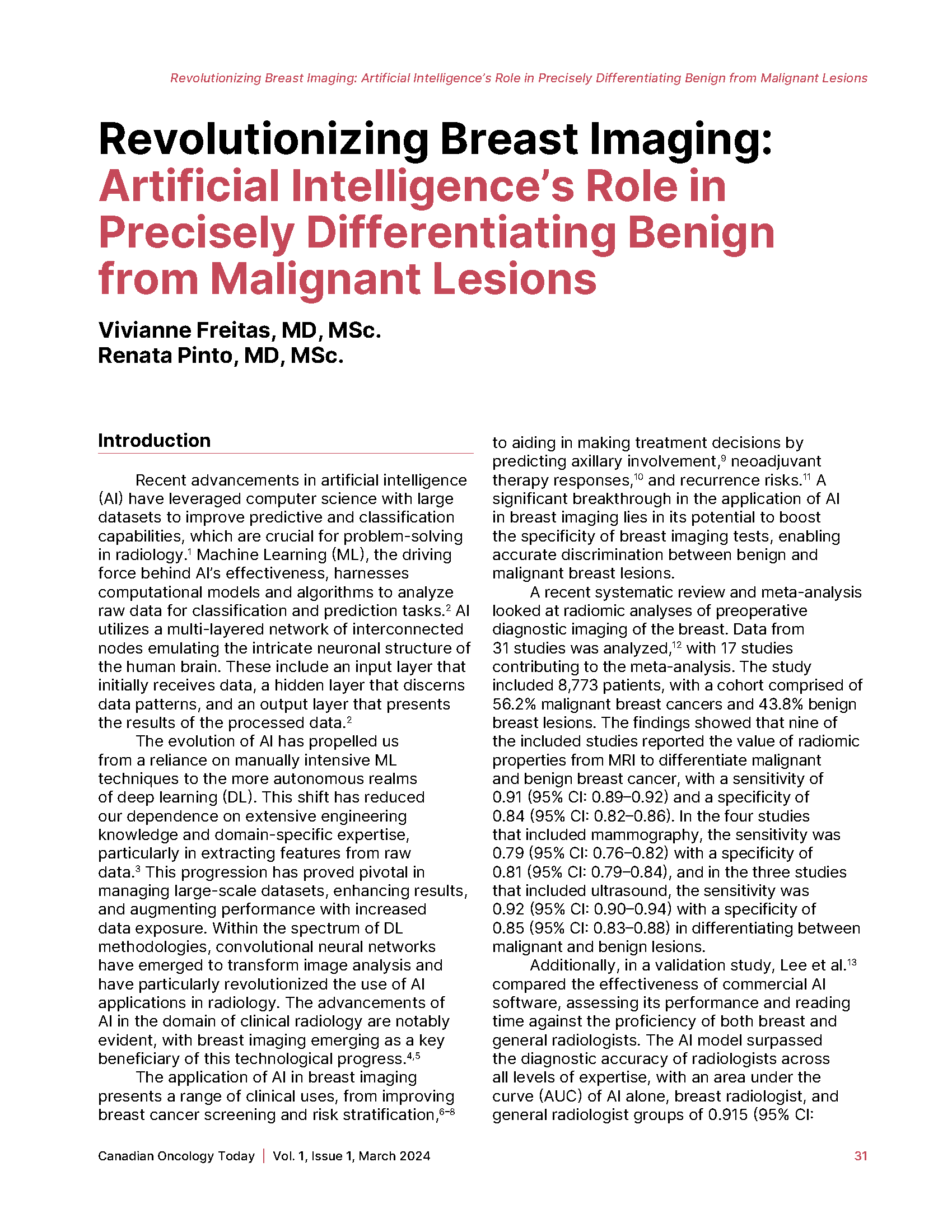
Published
How to Cite
Issue
Section
License
Copyright (c) 2024 Canadian Oncology Today

This work is licensed under a Creative Commons Attribution-NonCommercial-NoDerivatives 4.0 International License.